What is Survivorship Bias?
Survivorship bias is a type of bias that can occur in research studies when the focus is only on the successful outcomes while disregarding the unsuccessful ones. It happens when a researcher only studies the “survivors” or the people who have succeeded and ignores those who have failed or were excluded from the study. This approach can lead to a distorted understanding of the population being studied, as the sample size may not represent the entire population accurately.
For example, suppose a study focuses only on successful entrepreneurs. In that case, it may not be considering the factors that led to their failures, which could provide valuable insight and result in a biased conclusion. Survivorship bias can also be present in military studies. Only the planes that have survived are considered without considering those that have crashed, leading to insufficient protective measures and avoidable losses.
It’s essential to be aware of survivorship bias and to avoid it, such as considering the entire population being studied, not just those who have succeeded, and focusing on the process or factors that lead to success rather than just the outcome.
How Survivorship Bias Can Impact Research Studies
Survivorship bias can impact research studies in several ways, including:
- Incomplete data: Survivorship bias can result in incomplete or inaccurate data, as the sample size may not accurately represent the entire population being studied.
- False conclusions: If a researcher only studies those who have succeeded, they may draw incorrect conclusions about the factors that lead to success. This can lead to inaccurate recommendations or strategies.
- Missed opportunities: By only studying successful individuals or outcomes, a researcher may miss significant opportunities to learn from failures or mistakes.
What are Some Examples of Survivorship Bias?
If a researcher only studies those who have achieved success, they may miss significant opportunities to learn from failures or mistakes. There are many examples of survivorship bias across various fields. Here are a few examples:
- Military aircraft during World War II: During World War II, the US Air Force analyzed the bullet holes in returning planes to determine where to add additional armor. However, they only studied the aircraft that had survived their missions. This led to a bias towards reinforcing areas where planes were not hit rather than where they were hit. As a result, the additional armor was ineffective in protecting planes from the most common attack areas.
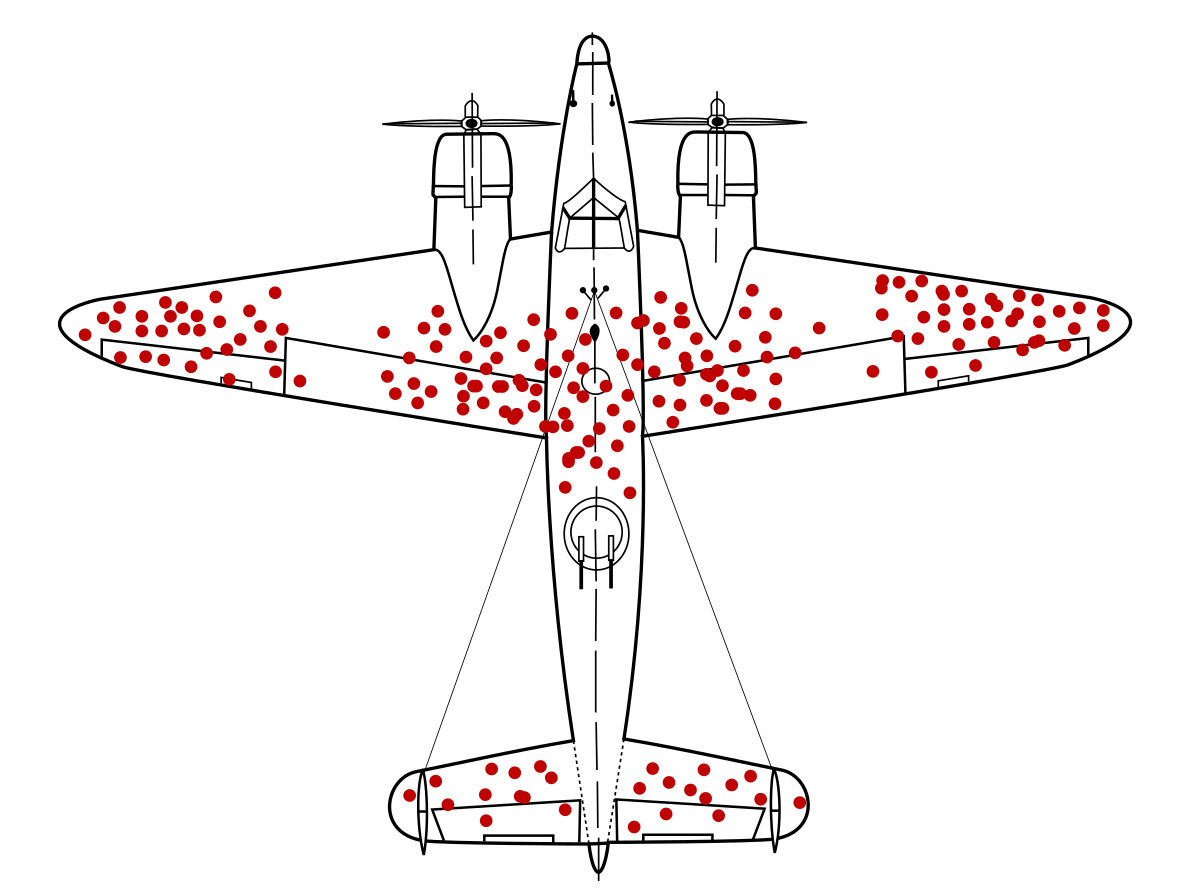
- Mutual fund performance: In the finance industry, survivorship bias can occur in the analysis of mutual fund performance. A mutual fund may be closed or merged with another fund if it performs poorly. This can lead to an overestimation of the performance of the remaining funds, as the underperforming funds are no longer included in the analysis.
- Entrepreneurship studies: In entrepreneurship studies, survivorship bias can occur when researchers only study successful entrepreneurs without considering those who have failed or dropped out. This can lead to an incomplete understanding of the factors that lead to success, as the sample size may not accurately represent the studied population.
- Medical research: Survivorship bias can also occur in medical research, such as in studies on cancer survival rates. If a study only looks at patients who have survived a certain amount of time, it may not consider the patients who have died early due to the disease or other factors. This can lead to overestimating survival rates and an incomplete understanding of the disease.
Practical Tips for Avoiding Survivorship Bias
To avoid survivorship bias in research studies, we recommend the following practical tips:
- Consider the entire population: When designing a research study, it is essential to consider the entire population being studied, not just those who have succeeded. This may involve including a representative sample of individuals who failed or were excluded from the study.
- Focus on the process, not just the outcome: Instead of only studying successful individuals or results, focus on the process or factors that lead to success. This may involve looking at successful and unsuccessful individuals to understand better the factors that lead to success.
- Use multiple data sources: To avoid relying solely on one, consider using various sources, including surveys, interviews, and secondary data sources.
- Be transparent about data collection: To ensure transparency and accuracy, be clear about the methods used to collect data, including any limitations or exclusions.
- Consult with experts: Consult with experts in the field to gain a more comprehensive understanding of the factors that may impact the study and to identify potential sources of bias.
Summary
Survivorship bias can harm research studies, leading to incomplete or inaccurate data, false conclusions, and missed opportunities. Suppose a researcher only studies those who have succeeded. In that case, it may result in an incomplete understanding of the studied population, as the sample size may not accurately represent the entire population.
Incomplete or inaccurate data can result from survivorship bias because the sample may not include those who failed or were excluded from the study. False conclusions may be drawn about the factors that lead to success because only successful outcomes were analyzed. Additionally, missed opportunities may occur because important data from unsuccessful results were not considered.
However, researchers can take practical steps to avoid survivorship bias and obtain a more comprehensive understanding of the population being studied. One way to prevent biased survivorship is by considering the entire population being studied, not just those who have succeeded. Researchers can also focus on the process or factors that lead to success, rather than just the outcome, to understand the factors at play better.
Using multiple data sources can also help avoid reliance on a single data source, which could lead to survivorship bias. Being transparent about data collection methods and limitations is essential to ensure accuracy and transparency in research studies.
Studies must consider out-of-sample data along with in-sample data to double-check their studies against information outside their control group. Focusing on only the research data with no external examples can lead to bias due to a small data set.