What is correlation?
Correlation is a statistical measure used to describe the relationship between two variables. When two variables are correlated, they are somehow related to one another. This connection could be positive, negative, or nonexistent.
A positive correlation is when an increase in one variable is associated with an increase in the other. For example, if you studied the relationship between stock prices and the overall sentiment on the economy, you might discover that as the belief in a strong economy grows, stock prices go higher. As the two variables move in the same direction, this is an example of a positive correlation.
Examples of positive correlation
Here are two examples of positive correlation:
- Increased exercise leads to weight loss: In this case, the amount of exercise someone does correlates positively with their weight; as the number of weight resistance exercises increases, so does their muscle mass. This is most likely because weight resistance exercises build muscles and can aid in the reduction of body fat.
- Higher education levels lead to higher salaries: In this case, an individual’s level of education is positively correlated with their salary. Their salary tends to rise in tandem with their level of education. Higher levels of education are likely to result in more specialized professional skills and knowledge, which can be valuable in the job market.
It’s critical to understand that correlation does not imply causation. A positive correlation between two variables does not always imply that one is causing the other. Other potential factors influencing the relationship between the two variables must be considered.
Identifying Positive Correlation
There are several ways to identify positive correlations in data. Here are two methods:
- Scatter plots: These are graphic representations of the relationship between two variables. It’s used to depict the magnitude and direction of the relationship between the variables. To make a scatter plot, plot the values of each variable on a graph, with one on the x-axis and the other on the y-axis. If the two variables have a positive correlation, the points on the scatter plot will form a line that slopes upward from left to right.
- Pearson’s correlation coefficient: A statistical measure used to describe the strength and direction of a relationship between two variables is Pearson’s correlation coefficient. It has a value between -1 and 1, with 1 indicating a strong positive correlation, 0 indicating no correlation, and -1 indicating a strong negative correlation. You will need data for variables and statistical software or a calculator to calculate Pearson’s correlation coefficient.
The strength of a positive correlation
The strength of a positive correlation refers to how closely the two variables are related to each other. Positive correlations can be weak, moderate, or strong.
A weak positive correlation means a slight relationship between the two variables but is not very strong. In other words, an increase in one variable is only slightly associated with an increase in the other variable. For example, there might be a weak positive correlation between the amount of time someone spends studying and their test scores. As the amount of time spent studying increases, their test scores might also slightly increase, but the relationship is not very strong.
A moderate positive correlation means a noticeable relationship between the two variables. An increase in one variable is moderately associated with an increase in the other variable. For example, there might be a moderate positive correlation between the amount of sleep someone gets and their productivity at work. As the number of sleep increases, their productivity might also increase, but not necessarily by a large amount.
A strong positive correlation means a very strong relationship between the two variables. An increase in one variable is strongly associated with an increase in the other variable. For example, there might be a strong positive correlation between the amount of exercise someone does and their weight. As the amount of exercise increases, their weight decreases significantly.
Limitations of correlation
It’s important to understand the limitations of correlation to interpret and analyze data accurately. Here are two limitations of correlation:
- Correlation does not imply causation: Just because two variables are correlated does not necessarily mean that one variable is causing the other. Correlation describes the relationship between two variables and does not prove that one variable is causing the other. It is important to consider other potential factors influencing the relationship between the two variables. For example, if there is a positive correlation between the amount of exercise someone does and their weight gain, it does not necessarily mean that the exercise is causing weight gain. Other factors, such as diet, calories, thyroid gland, or genetics, may influence the relationship.
- Other factors may influence the relationship: In addition to causation, other factors may influence the relationship between the two variables. These factors are known as confounding variables and can distort the relationship between the two variables being studied. For example, suppose you were studying the relationship between wearing a good luck charm and winning a game in sports. In that case, you might find a positive correlation between the two variables. However, other factors, such as weather, level of competition, and game readiness of the team, may influence the relationship between winning and the random nature of wearing a good luck charm. Consider these confounding variables to interpret the relationship between the two variables accurately.
Take these limitations into account when interpreting the results of a study or analysis that involves correlation. Understanding correlation’s limitations can help you accurately interpret and analyze data and draw meaningful conclusions from your findings.
Conclusion
Understanding positive correlation is essential for accurately analyzing and interpreting data. Positive correlation can aid in understanding the relationship between variables and be helpful in research and data analysis. However, it’s important to note that correlation does not imply causation and that other factors may influence the relationship between the two variables. You can improve your data interpretation and analysis skills by understanding the definition and characteristics of positive correlation and its limitations. All causes and effects must be considered over a large sample size to filter the noise from the signal.
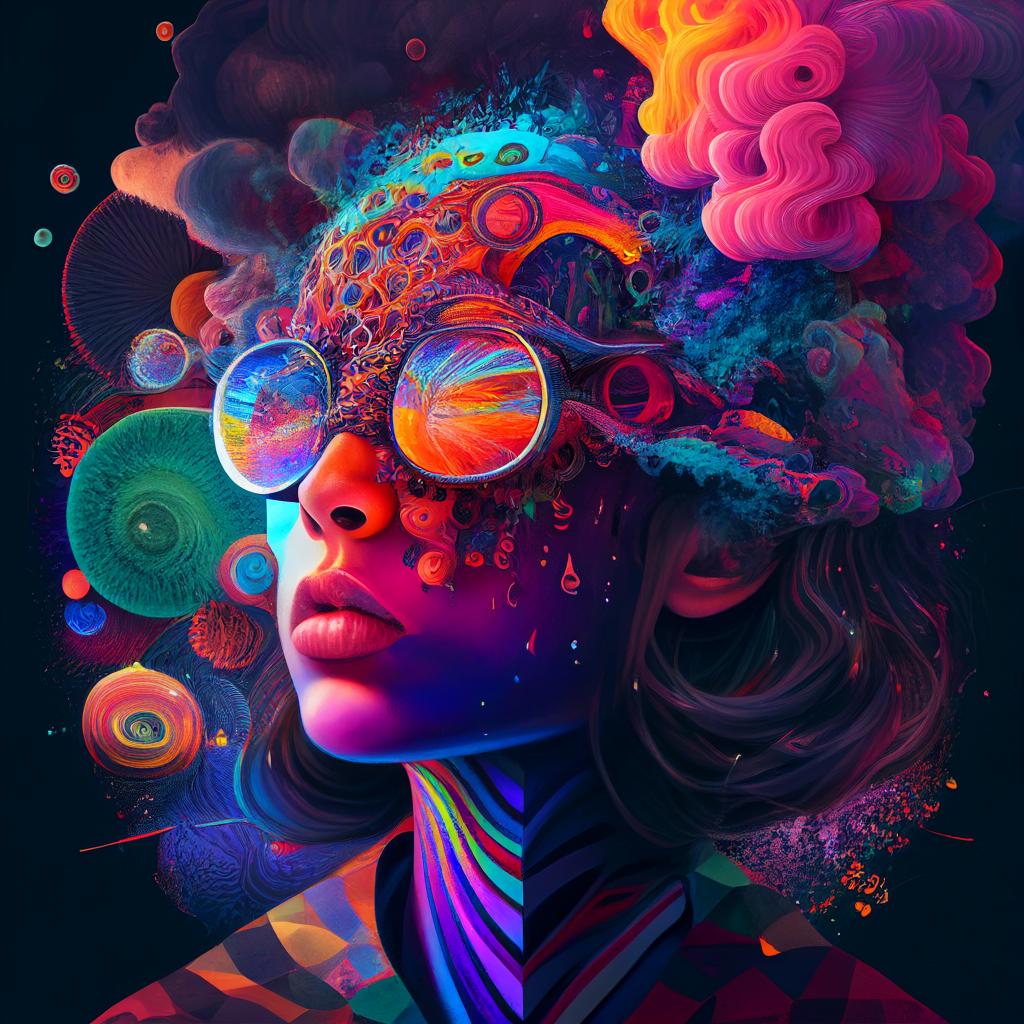